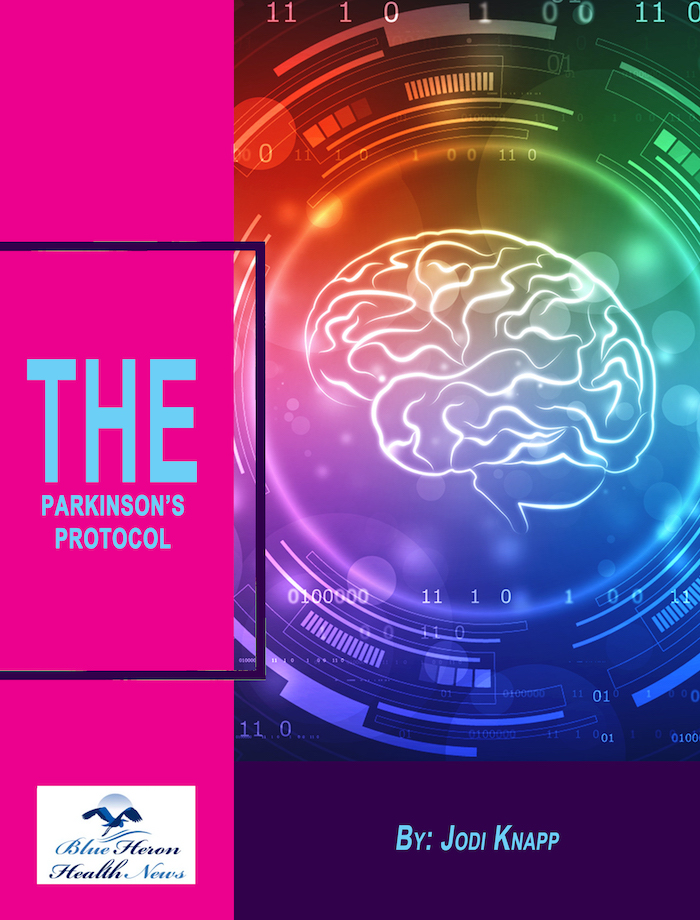
The Parkinson’s Protocol™ By Jodi Knapp Parkinson’s disease cannot be eliminated completely but its symptoms can be reduced, damages can be repaired and its progression can be delayed considerably by using various simple and natural things. In this eBook, a natural program to treat Parkinson’s disease is provided online. it includes 12 easy steps to repair your body and reduce the symptoms of this disease.
Machine Learning Applications in Parkinson’s Disease
Machine Learning (ML) is playing an increasingly important role in Parkinson’s Disease (PD) research and healthcare applications. ML algorithms can analyze complex data patterns to provide insights into disease diagnosis, progression, and treatment. Here are some key applications of machine learning in Parkinson’s Disease:
1. Early Diagnosis
- Movement Analysis: ML models can analyze video recordings or sensor data from wearables to detect subtle motor symptoms of Parkinson’s, such as tremors, bradykinesia (slowness of movement), and rigidity. These models can be used for early diagnosis, even before traditional clinical symptoms become obvious.
- Voice and Speech Analysis: PD affects speech patterns, often making voices softer, more monotone, or with slurred articulation. Machine learning algorithms can analyze voice recordings to detect changes in speech and provide early indicators of PD.
- Neuroimaging Data: ML is also applied to brain imaging techniques such as MRI and PET scans to identify characteristic changes in brain structure and function associated with PD. ML algorithms can detect early-stage changes in the brain, helping to identify PD long before symptoms are noticeable.
2. Predicting Disease Progression
- Machine learning models can analyze historical data from patients, such as age, symptoms, medication regimens, and brain scans, to predict how fast the disease will progress. These models can help personalize treatment plans and allow clinicians to better manage disease progression.
- Striatal Dopamine Imaging: ML can analyze dopamine transporter imaging (e.g., DaTscan) to predict the rate of dopamine loss in the brain, which is a key feature of PD progression.
3. Personalized Treatment
- Medication Management: ML algorithms can analyze how patients respond to medications over time, allowing for the optimization of treatment regimens. This can include adjusting dosages of levodopa (the primary medication for PD) or other medications to better manage symptoms.
- Side Effect Prediction: ML can predict which patients are more likely to experience side effects from specific treatments, allowing for more personalized and targeted care.
- Non-Motor Symptom Monitoring: PD affects not just motor functions but also non-motor symptoms such as sleep disorders, cognitive decline, and mood changes. ML can analyze data from patient surveys, wearables, and other health trackers to predict and manage non-motor symptoms.
4. Wearables and Remote Monitoring
- Continuous Monitoring: Wearables (such as smartwatches and accelerometers) can continuously track a PD patient’s movements, sleep patterns, and other physical activities. ML algorithms process this data to detect changes in the patient’s condition in real-time, facilitating remote monitoring and better management.
- Gait and Posture Analysis: ML can assess the gait (walking pattern) and posture of patients, which often changes with Parkinson’s. Analyzing this data helps in detecting early motor symptoms, tracking disease progression, and adjusting treatments.
5. Drug Discovery and Repurposing
- Identifying Potential Drug Candidates: ML is being used to analyze vast datasets, including genomic data, chemical properties of compounds, and clinical trial results. This can accelerate the identification of potential drug candidates that may benefit PD patients.
- Repurposing Existing Drugs: ML can also be used to find existing drugs (developed for other diseases) that may be effective for PD. By analyzing biological data and drug interactions, machine learning can help uncover new therapeutic applications for current medications.
6. Cognitive and Behavioral Analysis
- Parkinson’s Disease can lead to cognitive impairment, depression, and anxiety. Machine learning can analyze patient data such as cognitive tests, behavioral patterns, and even social media posts to detect early signs of cognitive decline or mood disorders, enabling early intervention.
- Dementia Prediction: ML can predict the risk of developing dementia in PD patients by analyzing cognitive scores, brain scans, and genetic data.
7. Clinical Trial Optimization
- Participant Selection: Machine learning can assist in identifying suitable participants for clinical trials based on their medical histories, genetic profiles, and disease stage. This helps ensure the right participants are selected, improving the chances of trial success.
- Real-Time Monitoring: During clinical trials, ML models can analyze real-time data to track the effectiveness of treatments and identify any adverse effects more rapidly.
- Data Integration: ML can process large datasets from diverse sources (genetic data, clinical assessments, biomarkers, etc.) and provide actionable insights that would be difficult for traditional methods to uncover.
8. Speech and Language Processing
- Speech Patterns as Biomarkers: ML models can be trained to detect early signs of Parkinson’s by analyzing voice features such as pitch, loudness, and speech rate. These speech patterns may be used as biomarkers for early-stage PD diagnosis or monitoring symptom progression.
- Speech Therapy Applications: ML-powered applications can help with speech therapy by analyzing patients’ vocal exercises and providing feedback, potentially improving the quality of life for individuals with PD who struggle with speech.
Challenges and Considerations
- Data Quality and Diversity: ML models require large amounts of high-quality data to train effectively. For Parkinson’s disease, this includes data from diverse patient populations, including different ages, genders, and ethnic backgrounds. Ensuring diversity in datasets helps improve the generalizability of models.
- Interpretability and Trust: Healthcare professionals must understand how ML models arrive at their decisions. It is crucial for clinicians to trust AI-driven recommendations, which requires the development of more interpretable and transparent ML models.
- Ethical and Privacy Concerns: Patient data must be handled securely and ethically, especially when using sensitive medical information. Ensuring data privacy and compliance with regulations (such as HIPAA) is essential when implementing ML in healthcare.
Conclusion
Machine learning is rapidly transforming Parkinson’s Disease research and clinical management. It enables early diagnosis, personalized treatments, efficient clinical trials, and the discovery of new drugs. As these technologies continue to evolve, they hold the potential to improve both the quality of life and life expectancy for Parkinson’s patients. However, ongoing research and ethical considerations are critical to fully realize the benefits of ML in PD.
The Parkinson’s Protocol™ By Jodi Knapp Parkinson’s disease cannot be eliminated completely but its symptoms can be reduced, damages can be repaired and its progression can be delayed considerably by using various simple and natural things. In this eBook, a natural program to treat Parkinson’s disease is provided online. it includes 12 easy steps to repair your body and reduce the symptoms of this disease.